

Sechidis, K., Tsoumakas, G., Vlahavas, I.: On the stratification of multi-label data.
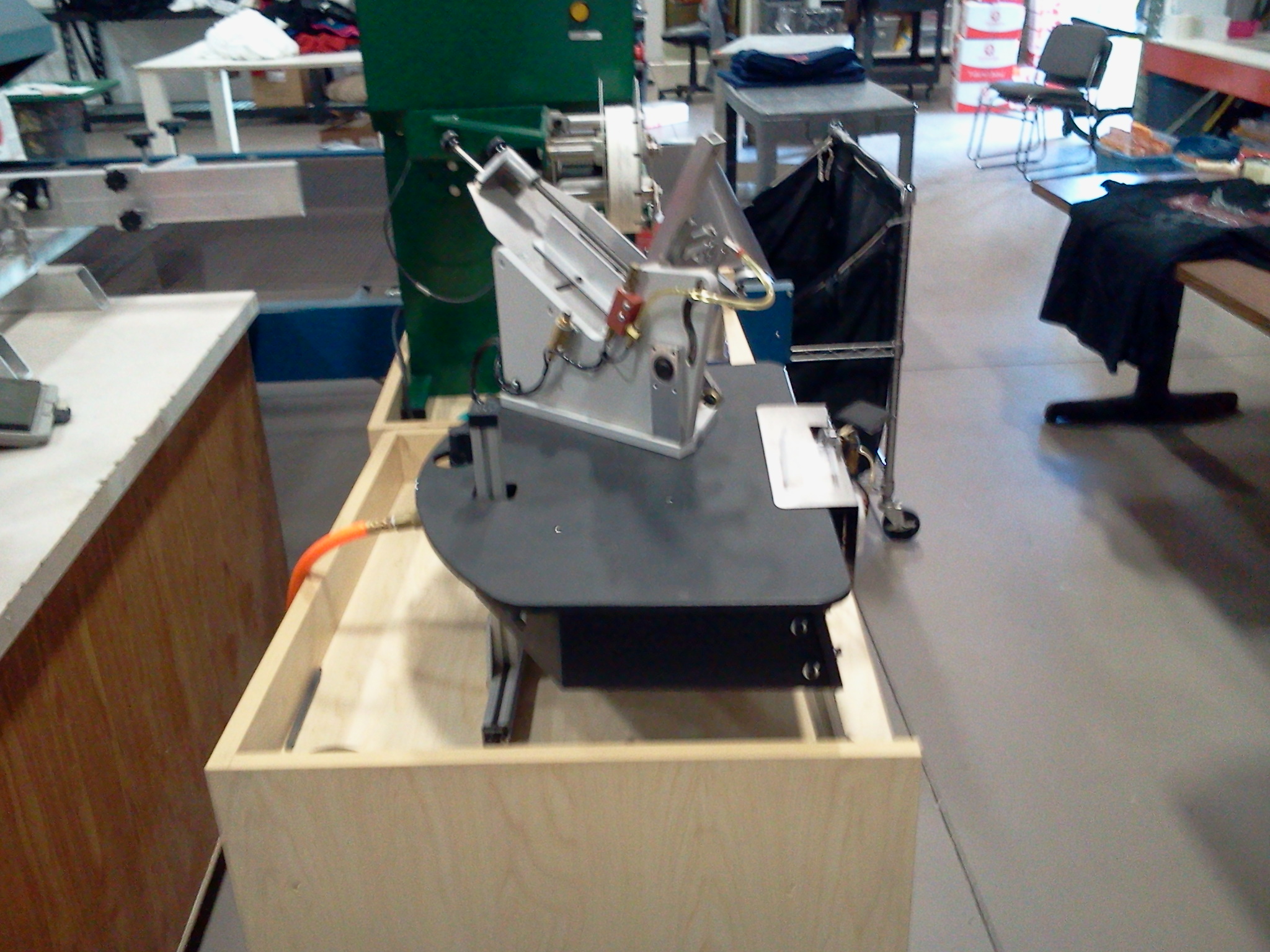
Spotify: Web API Reference | Spotify for Developers. Pedregosa FABIANPEDREGOSA, F., et al.: Scikit-learn: Machine Learning in Python Gaël Varoquaux Bertrand Thirion Vincent Dubourg Alexandre Passos Pedregosa, Varoquaux, Gramfort et al. Read, J., Pfahringer, B., Holmes, G., Frank, E.: Classifier chains for multi-label classification. Ĭhen, W.J., Shao, Y.H., Li, C.N., Deng, N.Y.: MLTSVM: A novel twin support vector machine to multi-label learning. īenites, F., Sapozhnikova, E.: HARAM: a hierarchical ARAM neural network for large-scale text classification. Zhang, M.L., Zhou, Z.H.: ML-KNN: A lazy learning approach to multi-label learning. Spyromitros, E., Tsoumakas, G., Vlahavas, I.: An empirical study of lazy multilabel classification algorithms. Szymański, P., Kajdanowicz, T.: A scikit-based Python environment for performing multi-label classification. Ibrahim, K.M., Epure, E.V., Peeters, G., Richard, G.: User-aware music auto-tagging with contextual tags (2020). Ibrahim, K.M., Epure, E.V., Peeters, G., Richard, G.: Contextual tags for music auto-tagging | Zenodo. Ibrahim, K.M., Royo-Letelier, J., Epure, E.V., Peeters, G., Richard, G.: Audio-based auto-tagging with contextual tags for music. Ĭhoi, K., Fazekas, G., Sandler, M.: Automatic tagging using deep convolutional neural networks. Won, M., Ferraro, A., Bogdanov, D., Serra, X.: Evaluation of CNN-based automatic music tagging models. Won, M., Spijkervet, J., Choi, K.: Music classification: beyond supervised learning. īogdanov, D., Won, M., Tovstogan, P., Porter, A., Serra, X.: The MTG-jamendo dataset for automatic music tagging. Ilarri, S., Trillo-Lado, R., Hermoso, R.: Datasets for context-aware recommender systems: current context and possible directions.
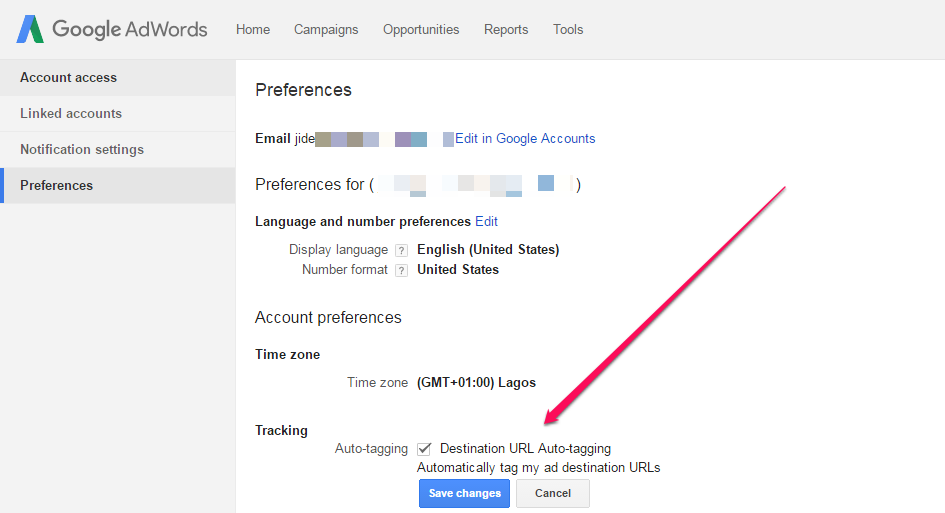
Sánchez-Moreno, D., Murciego, Á.L., López Batista, V.F., Dolores, M., Vicente, M., Moreno-García, M.N.: Dynamic inference of user context through social tag embedding for music recommendation (2021) Murciego, Á.L., et al.: Context-aware recommender systems in the music domain: a systematic literature review. Music tagging multi label classification.The results also point to the need for better representation of songs to use with these models to differentiate between closely related contexts. The results show how Spotify API features can be employed as simple features to use together with multi-label classification models to infer context labels for tracks. A comparison of different multi-label classification models is presented and discussed. In this paper we propose to infer activity context tags from simple song features obtained from the Spotify streaming service. Several works and datasets have been proposed in the literature to solve this problem. However, new songs added to the catalog or those belonging to the long tail do not have these tags and the need arises to create auto-taggers capable of tagging these songs. These tags are usually provided by users in social networks and are often present in popular songs in the catalog. Different types of tags have been used, such as those related to the musical genre, others related to the emotions evoked by the song, and others related to the user's context of action or activity. In the music domain, tags are commonly used to categorize the different songs a user listens to on streaming services such as Spotify or Deezer.
